E-Mail: | ibraheem(at)uni-koblenz.de |
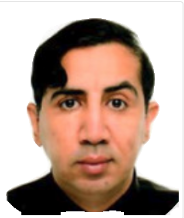
Dr. Ibraheem Al-Dhamari
About
Post doctoral researcher at the software engineering research group.
Research interests: Trustworthy AI, Deep Learning, Computer Vision, Medical Image Analysis, Data Privacy, and Cellular Automata
For international students: If you like to write your master or PhD thesis in our group and you need a scholarship, please check DAAD Scholarships, I will be happy to support you.
Web: https://uni-ko.de/ibraheem
Awards:
- DAAD full scholarships for MSc (2007), Short Research (2008), and PhD (2012).
External Profiles:
News
17.6.2024: Our paper, titled "Synthetic Datasets for Open Software Development in Rare Disease Research" has been accepted for publication in the Orphanet Journal of Rare Diseases.
2.5.2024: First day at my new position at the RGSE headed by Prof. Jürjens. Looking forward to the upcoming challenging tasks.
Projects
Current Projects:
- IH-evrsKI (2024-2025): Interdisciplinary hub for the provision of competencies in the development, handling, and application of explainable, trustworthy, resilient, and secure AI procedures. More info can be found here.
- PrivacyE2E (2024-2026): The project Privacy E2E aims at developing a framework that works data efficiently and only processes necessary information to both keep perfomance high and protect the end users’s privacy to the highest possible degree.
Completed Projects:
- EJP-RD (2021-2023): The European Joint Programme on Rare Diseases (EJP RD) brings together over 130 institutions from 35 countries to create a comprehensive, sustainable ecosystem for research, healthcare, and medical innovation in the field of rare diseases. As part of the project, I worked on tools and methods to support sustainable data exchange with a special focus on data protection aspects.
- Dynamic upper body surface volume measurement system (2019-2021): The aim of the project is to develop new patient movement analysis methods using dynamic and kinematic data from spine models.
- Personalized Expand (2017-2019): The goal of this project is the development of a customizable vertebra implant with a spongiosa surface texture.
- COMBS Project (2015-2017): The aim of this project is to develop new methods for clinical image analysis of the cochlea, including registration, fusion, and segmentation, as well as measurement of the volumes and varying lengths of the cochlear interstructures.
Publications
For an updated list please check my Google Scholar.
My PhD dissertation: Multi-modal 3D Cochlea Images Registration, Fusion, Segmentation and Analysis.
Journals:
- A l-Dhamari et al, (2024). Synthetic Datasets for Open Software Development in Rare Disease Research. Orphanet Journal of Rare Diseases (accepted).
- A l-Dhamari et al, (2023). Automatic Cochlea Multimodal 3D Image Segmentation And AnalysisUsing Atlas-model-based Method. Cochlea Implant International Journal, 2023,DOI:10.1080/14670100.2023.2274199.
- Waldeck et al, (2022). New ultra-fast algorithm for cochlear implant misalignment detection, European Journal of Radiology, Volume 151, 2022, 110283, ISSN 0720-0013,https://doi.org/10.1016/j.ejrad.2022.110283.
- Al-Dhamari et al, (2022). Automatic intra-subject registration and fusion of multimodal cochlea3D clinical images. PLOS ONE, 17(3), e0264449. https://doi.org/10.1371/journal.pone.0264449.
- Helal et al, (2021) Cone-beam CT versus Multidetector CT in Postoperative Cochlear ImplantImaging: Evaluation of Image Quality and Radiation Dose. American Journal Of Neuroradiology(AJNR),42(2021), Nr. 2, S. 362–367.
- Adwan et al (2013). Utilizing an enhanced cellular automata model for data mining". (2013)International Review on Computers and Software (IRECOS). 8 (2013), Nr. 2. S. 435-443.
- Dalhoum et al, (2012). Digital image scrambling using 2D cellular automata". (2012) IEEEMultimedia Journal. 19 (2012), Nr.4, S. 28-36.
Conferences:
- A l-Dhamari et al, (2019). Automatic Detection of Cervical Spine Ligaments Origin and InsertionPoints. IEEE 16 thInternational Symposium on Biomedical Imaging (ISBI 2019), 2019, S. 48–51.
- Al-Dhamari et al, (2018). Automatic Cochlear Length and Volume Size Estimation. In: Stoyanov,Danail et. al. (Hrsg.). OR 2.0 Context Aware Operating Theaters, Computer Assisted RoboticEndoscopy, Clinical Image Based Procedures, and Skin Image Analysis (MICCAI2018). SpringerInternational Publishing, 2018. ISBN 978–3–030–01201–4, S. 54–61.
- Al-Dhamari et al, (2018).Automatic Multimodal Cervical Spine Image Atlas Segmentation.Bildverarbeitung für die Medizin 2018. In-formatik aktuell. (2018) Springer Vieweg, Berlin, Heidelberg. S. 303-308.
- (Talk) Al-Dhamari (2017). Automatic Cochlea Segmentation Using Diffusion Snakes. 15thInternational Symposium on Cochlear Implants in Children, July 26–29, (2017), San Francisco,USA.
- (Talk) Al-Dhamari et al, (2017). ACIR: automatic cochlea image registration. Proceedings of SPIEMedical Imaging 2017, Image Processing, Orlando, USA. 10133 (2017), Nr. 10, S. 47-67.
- (Talk) Al-Dhamari (2016). Automatic Multimodal Registration and Fusion of 3D Human CochleaImages. 14 th International Conference on Cochlear Implants, May 11-14, (2016), Toronto, Canada.
- Dalhoum et al, (2010). fMRI brain data classification using cellular automata". (2010) Proceedingsof the 10 th international conference on applied informatics and communications. WorldScientific and Engineering Academy and Society (WSEAS,) S. 348-352.
- Sleit et al, (2010). An edge detection algorithm for online image analysis". (2010). Proceedings ofthe 2010 American conference on Applied mathematics, S.250-254.
- Sleit et al, (2010). An enhanced sub image matching algorithm for binary images. (2010)American Conf. on Applied Mathematics, S. 565-569.
- Sleit et al, (2009). Efficient enhancement on cellular automata for data mining. (2009)Proceedings of the 13 th WSEAS international conference on Systems, S. 616-620.
Theses
Current open topics:
- Trustworthy AI.
- 3D image translation, localization, instance segmentation, registration, and super-resolution.
- Synthetic Medical Data Generation.
- Data Privacy.
- Biomechanic simulation (with Dr. Sabine Bauer).
For Students: If you like to write research under my supervision, please send a cover letter, CV, and one-page research proposal.
Completed Theses:
Master:
- 2022, Li Ying Yin Simon, 3D Mesh Alignments Using Multi-resolution Non-rigid Image Registration Techniques
Bachelor:
- 2016, Robert Julien Claude Kohnen, Cochlea Spiral Shape Detection
Software
Selected open-source tools:
- ArxPy: a simple Python interface to Arx anonymization tool that supports DP.
- SynthMD: Synthetic Datasets for Software Development in Rare Disease Research.
- SlicerCochlea: 3D Slicer extension for cochlea registration fusion segmentation and analysis.
- SlicerCervicalSpine: 3D Slicer extension for cervical spine segmentation and landmarks detection.
About
Post doctoral researcher at the software engineering research group.
Research interests: Trustworthy AI, Deep Learning, Computer Vision, Medical Image Analysis, Data Privacy, and Cellular Automata
For international students: If you like to write your master or PhD thesis in our group and you need a scholarship, please check DAAD Scholarships, I will be happy to support you.
Web: https://uni-ko.de/ibraheem
Awards:
- DAAD full scholarships for MSc (2007), Short Research (2008), and PhD (2012).
External Profiles: