E-Mail: | zourlidou(at)uni-koblenz.de |
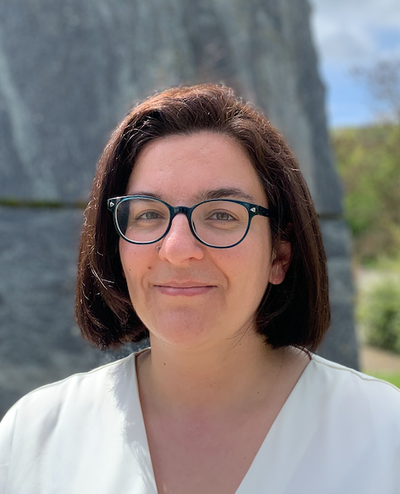
Dr. Ing. Stefania Zourlidou
I am a postdoctoral researcher and academic advisor (akademische Rätin) at the Institute for Web Science and Technologies (WeST) at the University of Koblenz. In this role, I teach and supervise master's theses on topics such as Machine Learning, Data Mining, Web Information Retrieval, and Network Theory. My current research focuses on the application of Artificial Intelligence in education, with the aim of enhancing learning experiences, improving student engagement, and developing personalized educational tools. I am passionate about leveraging AI to address educational challenges and create more effective and inclusive learning environments.
My research journey began with a strong emphasis on trajectory analysis and geospatial analysis. During my Ph.D. studies, under the supervision of Prof. Monika Sester, I worked on traffic regulation recognition using crowd-sensed data, contributing valuable insights to the field of geoinformatics. I hold a Ph.D. from the Institute for Cartography and Geoinformatics at Leibniz University Hannover. Additionally, I earned an M.Sc. in Intelligent Systems from University College London (U.K.), an M.A. in Educational Sciences from the Open University of Cyprus, an M.A. in Creative Writing from the University of Western Macedonia (Greece) and a B.Sc in Computer Science (Greece).
My multidisciplinary background allows me to bridge the gap between technology and education, and I am committed to advancing research at the intersection of these fields.
Research
My research interests span a diverse array of fields within Artificial Intelligence, Data Science, and Machine Learning, reflecting my academic and professional journey. In the past, I focused extensively on trajectory analysis and geospatial analysis, with significant contributions to traffic regulation recognition through the utilization of crowd-sourced data and geoinformatics. My work in this domain involved developing innovative methods for detecting and interpreting traffic regulations based on GPS trajectories and map-based inference, which has resulted in numerous publications and datasets that continue to support further research.
Currently, my research has evolved toward the application of AI in education. I am particularly interested in exploring how AI can enhance educational experiences, improve student engagement, and provide personalized learning opportunities. This shift aligns with the broader trends in AI, where the focus is increasingly on leveraging technology to solve real-world problems and create impactful solutions. Through my ongoing work, I aim to contribute to the development of intelligent systems that can adapt to the unique needs of learners, fostering a more inclusive and effective educational environment.
Teaching
Wint. Sem.’24-’25: AI-Driven Innovations in Education (Seminar)
Wint. Sem.’24-’25: Machine Learning and Data Mining (Lecture & Tutorial/Lab)
Summer Sem. ’24: Web Information Retrieval (Tutorial/Lab)
Summer Sem.’24: Network Theory and Dynamic Systems (Lecture & Tutorial/Lab)
Wint. Sem.’23-’24: Storytelling with Data (Seminar)
Wint. Sem.’23-’24: Machine Learning and Data Mining (Lecture & Tutorial/Lab)
Summer Sem.’23: Traffic Regulation Recognition from GPS Data (Seminar)
Summer Sem. ’23: Web Information Retrieval (Lecture & Tutorial/Lab)
Thesis Supervisions
The topics of the master's theses I supervise cover a broad spectrum of advanced subjects in AI, Data Science, and Machine Learning.
For students seeking thesis supervision: Before contacting me, please refer to the guide available on OLAT, which explains the entire process—from finding a thesis topic to submitting it to the examination office. I strongly recommend that students find their own topics, as this allows them to work on something they enjoy and are motivated by. However, if you have difficulty finding a topic, I can assist in formulating one that aligns with your interests. When reaching out, please include your most recent CV and a transcript of your studies.Open Thesis Topics:
Open Thesis Topics
1.AI-Driven Personalized Microlearning: Enhancing Student Skill Development Through Adaptive Bite-Sized Content Delivery (available from 01.2025)
The rapid evolution of educational technology has highlighted the need for personalized learning experiences that adapt to individual student needs. Microlearning, which delivers content in small, manageable chunks, is particularly effective for skill acquisition. However, the challenge lies in creating adaptive microlearning modules that respond dynamically to each student’s strengths and weaknesses. This research proposes to develop and evaluate AI-driven adaptive microlearning modules that optimize skill acquisition by personalizing content delivery for each learner.
Research Objectives
- To design AI-driven adaptive microlearning modules that tailor content to individual student needs.
- To evaluate the effectiveness of these modules in improving skill acquisition and retention compared to traditional learning methods.
- To assess student engagement and satisfaction with AI-driven adaptive microlearning.
2. EduCreativity: An AI-Powered Tool for Generating Creative Project Ideas Across Multiple Disciplines (availalable from 01.2025)
In today’s interdisciplinary educational landscape, the ability to generate creative and innovative project ideas is essential for fostering holistic learning. However, students often struggle to conceive original and cross-disciplinary projects due to limited exposure and resources. This research aims to develop and evaluate an AI-powered tool, EduCreativity, designed to generate creative project ideas tailored to students in various academic disciplines, enhancing interdisciplinary learning and innovation.
Research Objectives
- To develop an AI tool (EduCreativity) that generates creative and interdisciplinary project ideas based on user inputs from various academic disciplines.
- To evaluate the effectiveness of the EduCreativity tool in enhancing students' ability to develop innovative projects.
- To assess the impact of the tool on interdisciplinary collaboration and learning outcomes.
3. A Data-Driven Approach to Route Selection: Exploring Visualization Schemes for Aggregate Statistical Analysis of Urban Traffic Patterns (available from 01.2025)
Urban navigation poses significant challenges due to the complexity of traffic networks and the various factors that can influence travel efficiency. Drivers often have multiple route options between two points, each with unique characteristics such as the number of junctions, traffic lights, and average speed. Traditional navigation systems typically recommend routes based on criteria such as shortest distance or fastest estimated time of arrival (ETA). However, these recommendations often fail to account for other important factors that can affect the overall driving experience, such as the frequency of stops, wait times, and route consistency.
This thesis proposes a data-driven approach to route selection that aggregates various traffic metrics and explores innovative visualization schemes to present this information to users effectively. By evaluating not just the average travel time but also factors like the number of turns at junctions, number of traffic lights, average wait time at those lights, and other relevant statistics, this research aims to develop and evaluate different visualization techniques that can help users make more informed route decisions. Additionally, the research will explore the use of Large Language Models (LLMs) to automatically generate summary explanations of route comparisons based on these statistics.
Publications
S. Zourlidou, M. Sester, and S. Hu. Recognition of intersection traffic regulations from crowdsourced data. ISPRS International Journal of Geo-Information, 12(1), 2023.
S. Zourlidou, J. Golze, and M. Sester. Traffic regulation recognition using crowd-sensed gps and map data: a hybrid approach. AGILE: GIScience Series, 3:22, 2022.
S. Zourlidou, J. Golze, and M. Sester. [Dataset] traffic regulator ground-truth information of the city of Hannover, Germany, 2022.
S. Zourlidou, J. Golze, and M. Sester. [Dataset] Traffic regulator ground-truth information for the Chicago trajectory dataset, 2022.
S. Zourlidou, J. Golze, and M. Sester. [Dataset] GPS trajectory dataset of the region of Hannover, Germany, 2022.
S. Zourlidou, J. Golze, and M. Sester. [Dataset] gps trajectory dataset and traffic regulation information of the region of Edessa, Greece, 2022.
H. Cheng, H. Lei, S. Zourlidou, and M. Sester. Traffic control recognition with an attention mechanism using speed-profile and satellite imagery data. The International Archives of the Photogrammetry, Remote Sensing and Spatial Information Sciences, XLIII-B4-2022:287–293, 2022.
C. Wang, S. Zourlidou, J. Golze, and M. Sester. Trajectory analysis at intersections for traffic rule identification. Geo-spatial Information Science, 24(1):75–84, 2021, https://doi.org/10.1080/10095020.2020.1843374.
S. Zourlidou, J. Golze, and M. Sester. [Dataset] speed profiles and GPS trajectories for traffic rule recognition (6 junctions, Hannover, Germany), 2020.
J. Golze, S. Zourlidou, and M. Sester. Traffic regulator detection using GPS trajectories. KN - Journal of Cartography and Geographic Information, 2020.
H. Cheng, S. Zourlidou, and M. Sester. Traffic control recognition with speed-profiles: A deep learning approach. ISPRS International Journal of Geo-Information, 9(11), 2020.
S. Zourlidou and M. Sester. Traffic regulator detection and identification from crowdsourced data–a systematic literature review. ISPRS International Journal of Geo-Information, 8(11), 2019.
S. Zourlidou, C. Fischer, and M. Sester. Classification of street junctions according to traffic regulators. In Kyriakidis, P., Hadjimitsis, D., Skarlatos, D. &
Mansourian, A. (Eds.), 2019. Accepted Short Papers and Posters from the 22nd AGILE Conference on Geo–information Science. Cyprus University of Technology 17–20 June 2019, Limassol, Cyprus., June 2019.
M. Udahl, S. Zourlidou, and M. Sester. A spatiotemporal approach for detecting street intersections. In In: Kyriakidis, P., Hadjimitsis, D., Skarlatos, D. & Mansourian, A. (Eds.), 2019. Accepted Short Papers and Posters from the 22nd
AGILE Conference on Geo–information Science. Cyprus University of Technology 17–20 June 2019, Limassol, Cyprus., June 2019.
S. Zourlidou and M. Sester. Intersection detection based on qualitative spatial reasoning on stopping point clusters. In 23rd International Archives of the Photogrammetry, Remote Sensing and Spatial Information Sciences Congress, ISPRS 2016, 12–19 July 2016, Prague, Czech Republic. G¨ottingen: Copernicus GmbH, 2016.
C. Kuntzsch, S. Zourlidou, and U. Feuerhake. Learning the traffic regulation context of intersections from speed profile data. In In: Accepted Short Papers from the GIScience 2016 Workshop on Analysis of Movement Data (AMD’16), Montreal, Canada, 27 Sept, 2016.
S. Zourlidou and M. Sester. Towards regulation-aware navigation: a behavior-based mapping approach. In Accepted Short Papers from the 18th AGILE Conference on Geographic Information Science, Lisbon, Portugal., 2015.
S. Zourlidou and M. Sester. Road regulation sensing with in-vehicle sensors. In Ivanovic A. Comber, B. Bucher and S. (Eds.): Proceedings of the 3rd AGILE Phd School, Champs sur Marne, France, 15–17 September 2015, published at http://ceur-ws.org, 2015.
M. Sester, U. Feuerhake, C. Kuntsch, and S. Zourlidou. Interpretation of moving point trajectories. In Photogrammetric Week 15, Stuttgart, Germany., 2015.
S. Zourlidou, G. Stylios, and G. Giakas. Processing gait analysis data: A software system for estimating muscle variations. In 3rd European medical and biological engineering and IFMBE conference (EMBEC’05), 2005.
F. Perez-Cruz, S. Zourlidou, and Z. Ghahramani. Dirichlet prior for Bayesian GARCH. In Workshop on Machine Learning in Finance at NIPS’05, 2005
S. Zourlidou, G. Stylios, and G. Giakas. A software system for the estimation of the quadricepts variations using a gait analyis system. In 4th European Symposium in Biomedical Engineering, 2004.
HiWi Jobs
Currently, I do not have any Hiwi job openings available for the upcoming Winter Semester 2024/2025.
Typically, for my teaching activities, I employ one student assistant to help with the lectures/tutorials/exams of my courses. If you are interested in applying for future semesters, please ensure that your application is submitted (email me) at least 2-3 months before the semester begins. The application should include your current transcript and CV. A strong grade in the course you wish to assist with is highly desirable and will be considered a significant advantage.
I am a postdoctoral researcher and academic advisor (akademische Rätin) at the Institute for Web Science and Technologies (WeST) at the University of Koblenz. In this role, I teach and supervise master's theses on topics such as Machine Learning, Data Mining, Web Information Retrieval, and Network Theory. My current research focuses on the application of Artificial Intelligence in education, with the aim of enhancing learning experiences, improving student engagement, and developing personalized educational tools. I am passionate about leveraging AI to address educational challenges and create more effective and inclusive learning environments.
My research journey began with a strong emphasis on trajectory analysis and geospatial analysis. During my Ph.D. studies, under the supervision of Prof. Monika Sester, I worked on traffic regulation recognition using crowd-sensed data, contributing valuable insights to the field of geoinformatics. I hold a Ph.D. from the Institute for Cartography and Geoinformatics at Leibniz University Hannover. Additionally, I earned an M.Sc. in Intelligent Systems from University College London (U.K.), an M.A. in Educational Sciences from the Open University of Cyprus, an M.A. in Creative Writing from the University of Western Macedonia (Greece) and a B.Sc in Computer Science (Greece).
My multidisciplinary background allows me to bridge the gap between technology and education, and I am committed to advancing research at the intersection of these fields.
UNIVERSITY OF KOBLENZ
Universitätsstraße 1
56070 Koblenz